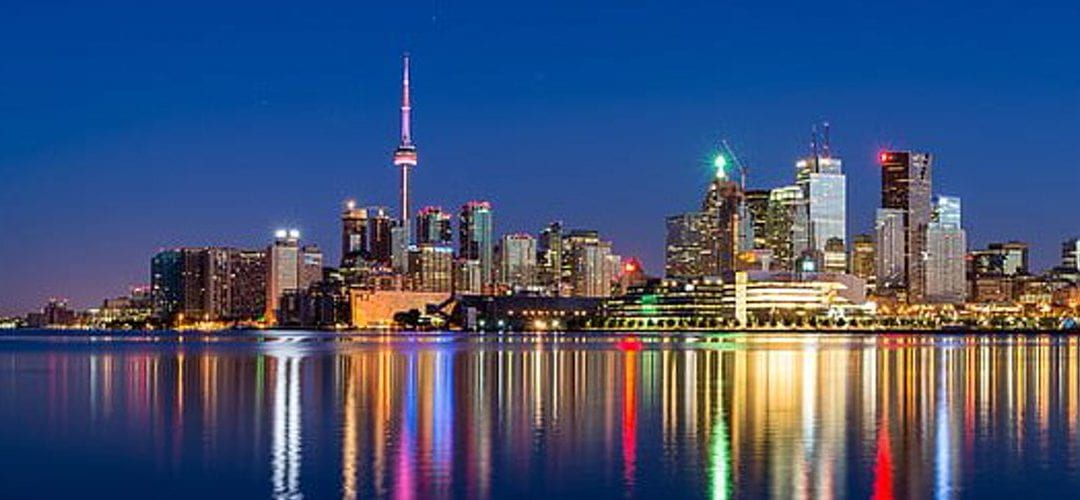
UCSC Goes to ACL 2023
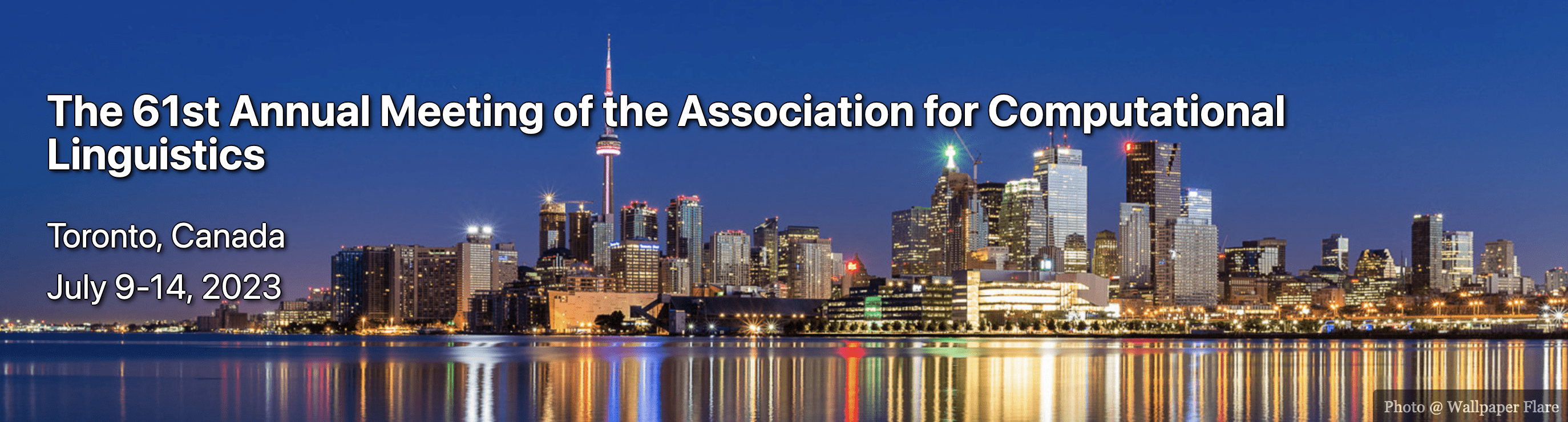
It’s a fascinating time to work in natural language processing. In recent years, we’ve witnessed incredible advances in AI and a growing global interest in the NLP methods and technologies driving recent innovations. This year, industry experts and research scientists gathered in Toronto at the 61st Annual Meeting of the Association for Computational Linguistics to discuss the field’s emerging trends and developments, and to showcase important contributions to state-of-the-art research in NLP, computational linguistics, and machine learning.
We’re proud to share that UCSC faculty and students presented six papers at the conference. Keep reading to learn more about each paper and the high-impact research conducted by UCSC’s NLP community.
CausalDialogue: Modeling Utterance-level Causality in Conversations
Yi-Lin Tuan, Alon Albalak, Wenda Xu, Michael Saxon, Connor Pryor, Lise Getoor, William Yang Wang
Abstract preview: Despite their widespread adoption, neural conversation models have yet to exhibit natural chat capabilities with humans. In this research, we examine user utterances as causes and generated responses as effects, recognizing that changes in a cause should produce a different effect. To further explore this concept, we have compiled and expanded upon a new dataset called CausalDialogue through crowd-sourcing. Read the full abstract and paper in the ACL Anthology.
Aerial Vision-and-Dialog Navigation
Yue Fan, Winson Chen, Tongzhou Jiang, Chun Zhou, Yi Zhang, Xin Wang
Abstract preview: The ability to converse with humans and follow natural language commands is crucial for intelligent unmanned aerial vehicles (a.k.a. drones). It can relieve people’s burden of holding a controller all the time, allow multitasking, and make drone control more accessible for people with disabilities or with their hands occupied. To this end, we introduce Aerial Vision-and-Dialog Navigation (AVDN), to navigate a drone via natural language conversation. Read the full abstract and paper in the ACL Anthology.
Automatic Identification of Code-Switching Functions in Speech Transcripts
Abstract preview: Code-switching, or switching between languages, occurs for many reasons and has important linguistic, sociological, and cultural implications. Multilingual speakers code-switch for a variety of communicative functions, such as expressing emotions, borrowing terms, making jokes, introducing a new topic, etc. The function of code-switching may be quite useful for the analysis of linguists, cognitive scientists, speech therapists, and others, but is not readily apparent. To remedy this situation, we annotate and release a new dataset of functions of code-switching in Spanish-English. Read the full abstract and paper in the ACL Anthology.
Diverse Retrieval-Augmented In-Context Learning for Dialogue State Tracking
Brendan King, Jeffrey Flanigan
Abstract preview: There has been significant interest in zero and few-shot learning for dialogue state tracking (DST) due to the high cost of collecting and annotating task-oriented dialogues. Recent work has demonstrated that in-context learning requires very little data and zero parameter updates, and even outperforms trained methods in the few-shot setting. We propose RefPyDST, which advances the state of the art with three advancements to in-context learning for DST. Read the full abstract and paper in the ACL Anthology.
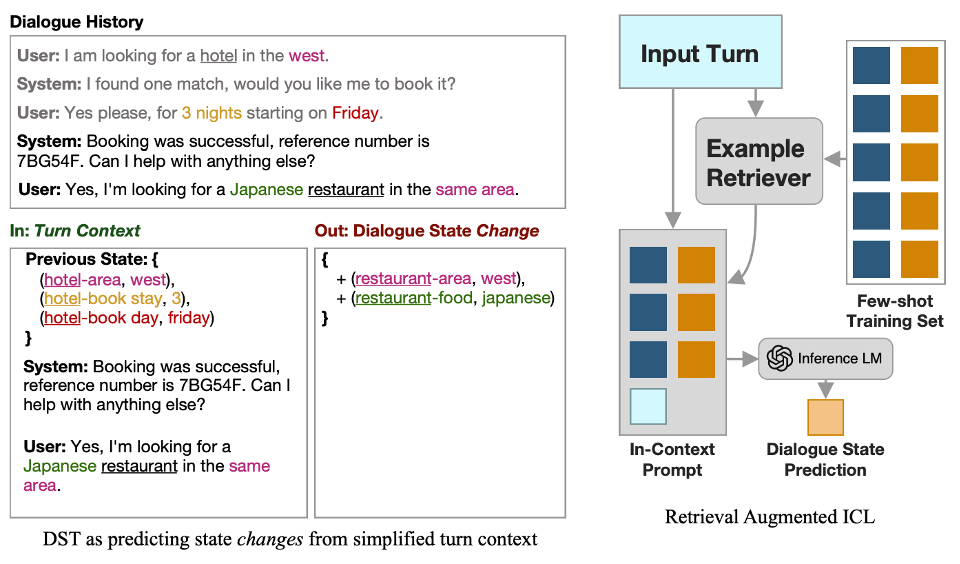
ACL 2023 poster prepared by Brendan King and NLP faculty member, Professor Jeffrey Flanigan, showcasing their paper, “Diverse Retrieval-Augmented In-Context Learning for Dialogue State Tracking”
T2IAT: Measuring Valence and Stereotypical Biases in Text-to-Image Generation
Jialu Wang, Xinyue Liu, Zonglin Di, Yang Liu, Xin Wang
Abstract preview: *Warning: This paper contains several contents that may be toxic, harmful, or offensive.* In the last few years, text-to-image generative models have gained remarkable success in generating images with unprecedented quality accompanied by a breakthrough of inference speed. Despite their rapid progress, human biases that manifest in the training examples, particularly with regard to common stereotypical biases, like gender and skin tone, still have been found in these generative models. In this work, we seek to measure more complex human biases existing in the task of text-to-image generations. Inspired by the well-known Implicit Association Test (IAT) from social psychology, we propose a novel Text-to-Image Association Test (T2IAT) framework that quantifies the implicit stereotypes between concepts and valence, and those in the images. Read the full abstract and paper in the ACL Anthology.
Using Domain Knowledge to Guide Dialog Structure Induction via Neural Probabilistic Soft Logic
Connor Pryor, Quan Yuan, Jeremiah Liu, Mehran Kazemi, Deepak Ramachandran, Tania Bedrax-Weiss, Lise Getoor
Abstract preview: Dialog Structure Induction (DSI) is the task of inferring the latent dialog structure (i.e., a set of dialog states and their temporal transitions) of a given goal-oriented dialog. It is a critical component for modern dialog system design and discourse analysis. Existing DSI approaches are often purely data-driven, deploy models that infer latent states without access to domain knowledge, underperform when the training corpus is limited/noisy, or have difficulty when test dialogs exhibit distributional shifts from the training domain. This work explores a neural-symbolic approach as a potential solution to these problems. Read the full abstract and paper in the ACL Anthology.
Congratulations to all who presented at the 2023 ACL Conference! We are tremendously proud of our UCSC faculty and students for their important contributions to high-impact research in NLP, AI, and machine learning.